Social and Structural Inequalities and COVID-19 in the United States
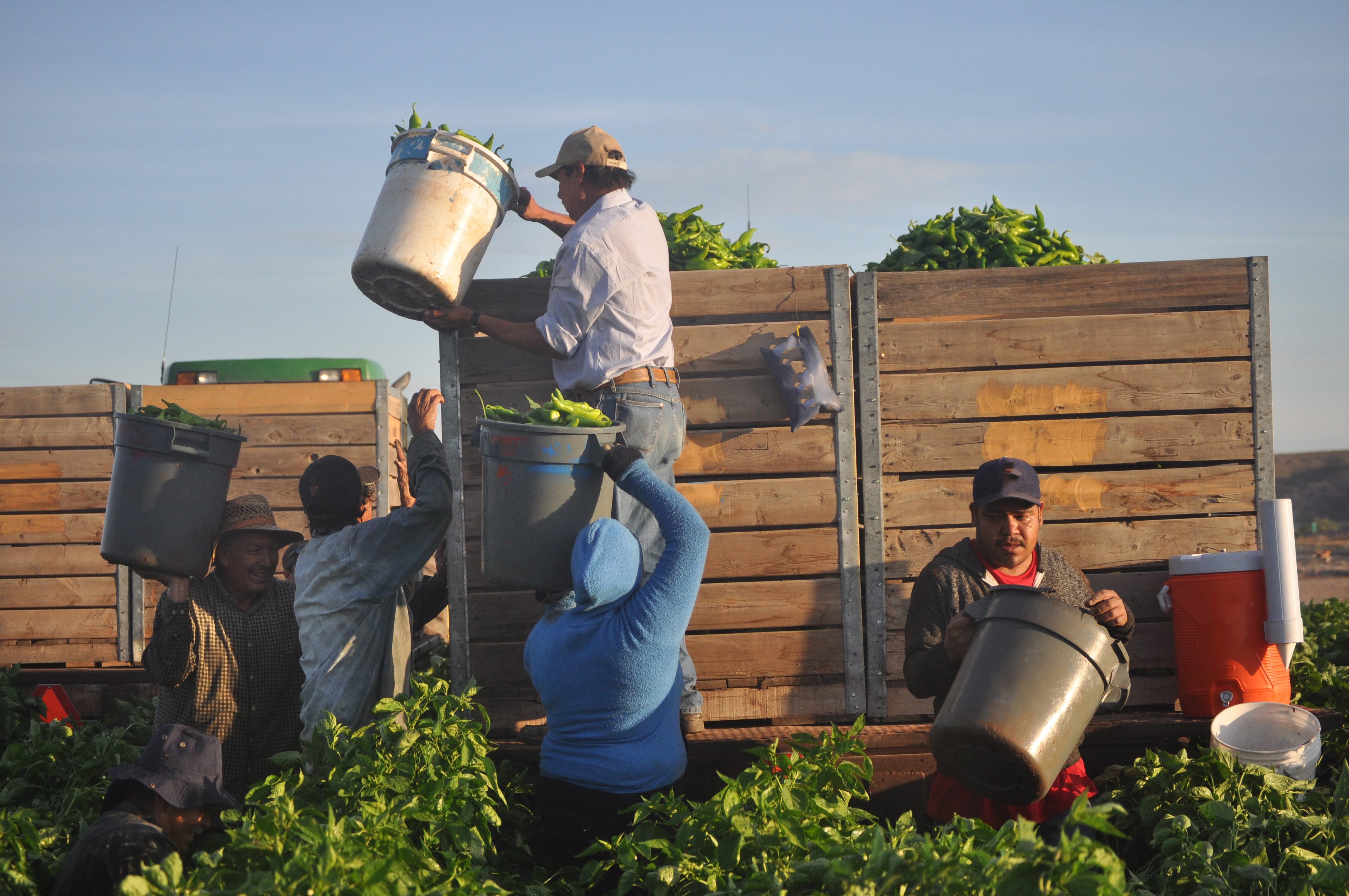
Introduction
The current pandemic of severe acute respiratory syndrome caused by the novel coronavirus SARS-COV-2 started in Wuhan (Hubei, China), in December 2019 and created a worldwide outbreak of the disease COVID-19. On January 20, 2020, the first case was confirmed in Snohomish County, Washington in the United States. As of August 31, 2020, a cumulative total of 5,997,636 COVID-19 cases (Figure 1) and 182,169 deaths were reported in the United States (Figure 2) and continued to increase. The cumulative number of COVID-19 cases and deaths vary significantly by state, metropolitan/nonmetropolitan areas, and counties. New York metropolitan area was the epicenter at the beginning of the pandemic, but growth in cases and deaths were reported in other large metropolitan areas such as New Orleans, Chicago, Milwaukee, and Detroit and in smaller ones like Albany, GA, and later in many rural areas in the South and other regions.
The coronavirus pandemic has exposed and exacerbated the already existing social, structural, and spatial inequalities in the country and their implications on health disparities. Minority populations, especially Latino and African American populations, were disproportionately contracting SARS-COV-2 at high rates and were more likely to die from COVID-19. As of September 8, 2020, according to the Centers for Disease Control and Prevention1, of persons confirmed to have contracted COVID-19 and for whom race/ethnicity was available, 30.3% were Latinos, 19.0% were Blacks, 41.2% were Whites, 3.5% were Asians, and 1.2% were American Indians/Alaska Natives. Of those who died from COVID-19, and for whom race/ethnicity was available, 21.9% were Blacks, 16.7% were Latinos, 51.2% were Whites, 5.0 were Asians, and 0.8% were American Indians/Alaska Natives.2 Racial and socioeconomic disparities in COVID-19 cases and deaths, especially in predominantly minority communities suggest that race/ethnicity, social class, and structural and spatial inequalities contribute to COVID-19.
Conceptually, this study relies on the framework by the World Health Organization (WHO) Committee on Social Determinants of Health (CSDH) for understanding the disproportionate social structural inequalities in COVID-19 cases and deaths. The CSDH framework highlights the upstream social, physical, and economic environments as the foundation from which health outcomes and health disparities arise. This framework recognizes the unequal distribution of power, income, goods, and services, globally and nationally, the consequent unfairness in the immediate, visible circumstances of people’s lives—their access to health care and education, their conditions of work and leisure, their homes, communities, towns, or cities—and their chances of leading a flourishing life. This unequal distribution of health-damaging experiences is not in any sense a natural phenomenon but is a result of a combination of social policies and programs, unfair economic arrangements, and politics. Together, the structural determinants and conditions of daily life constitute the social determinants of health and cause much of the health inequality between and within countries. We postulate that the disproportionate nature of COVID-19 cases and deaths are a result of existing social and structural inequalities, especially in a context in which there has not been a coordinated national response.
This study examines the relationship between social structural inequalities and COVID-19 cases and deaths at the county level. We analyze cross-sectional associations between concentrated disadvantage, income inequality, racial/ethnic composition, and immigration concentration and COVID-19 cases and deaths, controlling for potential confounders, including chronic disease comorbidities, proportion of uninsured residents, proportion of residents 65 years or older, residential mobility, and metropolitan location.
Background and Significance
The impact of COVID-19 goes well beyond the viral infection itself, reflecting the dynamics of long-standing adverse host environments characterized by institutional processes that produce limited access to resources (i.e., money, food, education, health care, job flexibility), making disadvantaged communities more vulnerable during the pandemic. It is commonly known that greater social disadvantage in educational attainment and income is associated with poorer health in both the United States and Europe. According to Williams and Cooper (2020), the striking racial/ethnic disparities reported for COVID-19 infection, testing, and disease burden are a clear reminder that failure to protect the most vulnerable members of society not only harms them, but also increases the spread of the virus with devastating health and economic consequences for all. COVID-19 disparities are not the fault of the most vulnerable populations, instead they reflect social policies and systems that maintain health disparities in good times and inflate them during a crisis. While the coronavirus does not discriminate, systemic processes point to the likelihood that socially disadvantaged groups would have higher rates of COVID-19 confirmed cases and deaths. People from low socioeconomic backgrounds, those located in the secondary labor market, and racial and ethnic minority populations concentrated there, are unlikely to have the necessary financial and other resources to make self-distancing and self-isolation a viable option within the context of their daily lives.
Physical and service characteristics of neighborhoods can create and reinforce socioeconomic and racial/ethnic disparities in health. Latinos and African Americans, for example, live in residentially segregated neighborhoods, and this has consequences in the areas of education, occupations, and health. Do and colleagues (2017) found that segregation has negative effects on the health of U.S.-born Latinos.3 Similarly, Kim and Bostwick (2020) found that COVID-19 disproportionately affected poor, highly segregated African American communities in Chicago. They found significant spatial clusters of social vulnerability and risk factors, both of which are significantly associated with increased COVID-19-related death rates. They also found that a higher percentage of African Americans was associated with increased levels of social vulnerability and risk factors. In addition, they found that the proportion of African American residents has an independent effect on the COVID-19 death rate and that the disproportionate effects of COVID-19 in African American communities are a reflection of racial inequality and social exclusion that existed before the COVID-19 crisis.4
Wadhera and colleagues (2020) also show that risks associated with COVID-19 varied markedly by borough of residence in New York City. The Bronx had the lowest levels of income and education and the highest proportion of Latino and Black residents and the highest rate of COVID-19 hospitalizations and deaths, whereas Manhattan, the predominantly White and most affluent borough of New York City, had the lowest rate of hospitalizations and deaths.5 Yancy (2020) also found that in Chicago, 50 percent of COVID-19 cases and nearly 70 percent of COVID-19 deaths involve Black individuals, although Blacks make up only 30 percent of the city population. Moreover, these deaths were concentrated mostly in just five neighborhoods.6
Race/ethnicity remains an important social factor that influences health primarily because of racism. The effects of racism occur primarily through deep-seated societal structures that systematically constrain opportunities and resources for ethno-racial populations that historically have been the targets of racism by White American institutions. Racial residential segregation, in particular, is a key mechanism through which racism produces and perpetuates social disadvantage.7 Latinos and Blacks are more likely to reside in disadvantaged neighborhoods with inadequately resourced schools characterized by low quality educational processes and low educational attainment levels by students, with resultant health effects. The highest concentrations of lead in Flint, for example, were located in its most disadvantaged neighborhoods.
Latinos and African Americans and many people of color are more likely than White Americans to provide high-risk essential services, including low-wage jobs that cannot be done remotely. They also tend to have fewer financial resources to draw on in the event of health problems or economic disruption. Further, underlying comorbidity conditions such as cardiovascular disease, hypertension, diabetes, obesity, and asthma reflect structural societal flaws beyond simply poor lifestyle choices and ultimately lead to higher COVID-19 mortality rates in both rural and urban environments. Ethno-racial minorities are more likely to be uninsured compared to non-Hispanic Whites, and uninsured adults are less likely to have control over cardiovascular disease risks. These comorbidity conditions that make COVID-19 more deadly are linked to segregation and concentrated poverty.
Racism affects health more directly through pathways involving stress. Chronic stress related to experiences of racism, including relatively subtle experiences arising even without consciously prejudicial intent, may contribute to racial/ethnic disparities in health, regardless of one’s neighborhood, income, or education.8 Whitney (2020) argues that racism and capitalism construct harmful social conditions that fundamentally shape COVID-19 disease inequities because they shape multiple diseases that interact with COVID-19 to influence poor health outcomes; affect disease outcomes through increased multiple risk factors for poor people of color, including racial residential segregation, homelessness, and medical bias; shape access to flexible resources, such as medical knowledge and freedom, which can be used to minimize both risks and the consequences of disease; and replicate historical patterns of inequities within pandemics, despite newer intervening mechanisms thought to ameliorate health consequences.9
Data and Methods
Data were drawn from publicly available secondary sources, including USAFacts cumulative COVID-19 cases and deaths data by county through September 8, 2020; the CDC Surveillance System for the prevalence of diabetes and obesity; the CDC Interactive Atlas of Heart Disease and Stroke (2016-2018); and the U.S. Census Bureau 2013-2018 American Community Survey (ACS) summary files for county sociodemographic characteristics downloaded from IPUMS NHGIS, University of Minnesota.10
Table 1 displays descriptive statistics for all major variables. The outcome variables are COVID-19 confirmed cases and COVID-19 deaths. The mean number of confirmed COVID-19 cases by county was 1,567.40 (standard deviation [SD] = 6,999.79) and the mean number of COVID-19 deaths was 50.91 (SD = 295.02), respectively. The mean county COVID-19 infection rate per 100,000 people was 1,094.12 cases (SD = 1,085.19). The mean county COVID-19 mortality rate per 100,000 people was 50.91 (SD = 295.02).
Table 1. Descriptive Statistics of Selected Characteristics (N = 3,140), February – September 2020
We used concentrated disadvantage index, Gini index, racial/ethnic and immigrant concentrations to measure county-level social inequality. Concentrated disadvantage index is a principal component factor that linearly combines eight variables: poverty, unemployment, receipt of public assistance, female-headed families, percentage of residents 25 years and older with less than a high school education, percentage of residents 25 years and older with a Bachelor degree or higher, percentage of affluent households (≥ $75,000), and percentage of managerial and professional occupations. Each variable is weighted by its factor loading. The first five variables had positive factor loadings whereas the reminders had negative factor loadings (See Appendix A). Higher values on this score indicate counties with a high concentration of disadvantage. The concentrated disadvantage score ranges from -15.70 to 15.09 with a mean of 0 (SD = 3.98) (Table 1).
Income inequality was measured using the Gini index. The Gini index has a possible range between 0 and 1 where 0 represents complete income equality and 1 represents maximum income inequality. Income inequality ranges from 0.26 to 0.67 with a mean of 0.45 (SD = 0.04).
Racial/ethnic composition and immigrant concentration. The county racial/ethnic makeup was measured by the percentages of Latino, African American, Asian, and Native American residents. The percentage of Latino residents ranged from 0 to 99.07 percent with a mean of 9.27 percent (SD = 13.79). The percentage of African American residents ranged from 0 to 87.41 percent with a mean of 8.93 percent (SD = 14.47). The percentage of Asian residents ranged from 0 to 53.33 percent with a mean of 1.44 percent (SD = 3.08). The percentage of Native American residents ranged from 0 to 82.48 percent with a mean of 1.77 percent (SD = 7.22). The county immigrant concentration was measured by the percentage of foreign-born residents. The percentage of immigrant residents ranged from 0 to 53.25 percent with a mean of 4.73 percent (SD = 5.71).
Covariates. This study includes a set of covariates to control for potential confounding of county effects on COVID-19 cases and deaths. The covariates include the percentage of residents 65 years and older, the percentage of residents who have moved in the last five years, metropolitan residence, the percentage of residents without health insurance coverage, an index of chronic disease comorbidities, a standardized factor score that combines the percentage of adult residents aged 20 years and older who are classified as obese (BMI ≥30), the percentage of adult residents aged 20 years and older with diabetes, and cardiovascular disease, stroke, and hypertension death rates per 100,000 people 35 years and older.
The percentage of residents 65 years and older ranged from 3.80 percent to 55.60 percent with a mean of 18.37 percent (SD = 4.58 percent). The percentage of residents who have moved in the last five years ranged from 5.22 percent to 51.89 percent with a mean of 16.78 percent (SD = 3.81 percent). The percentage of residents with no health insurance coverage ranged from 1.74 percent to 45.59 percent with a mean of 10.07 percent (SD = 5.08 percent). About 37.1 percent of counties (1,166/3,140) were in metropolitan areas and the remaining counties were in nonmetropolitan areas.
The chronic disease index ranges from -3.72 to 4.72 with a mean of 0 (SD = 1). One third of residents (33.43%) were considered obese (BMI ≥ 30). The obesity prevalence ranged from 11.0 percent to 58.9 percent. The diabetes prevalence ranged from 2.2 percent to 28.7 percent, with a mean of 10.49 percent (SD = 3.52). The mean cardiovascular disease (CVD) death rate was estimated at 465.21 per 100,000 people (SD = 98.91), ranging from 93.8 to 1,028 CVD deaths per 100,000 people. The mean stroke death rate was estimated at 77.10 per 100,000 people (SD = 16.22), ranging from zero to 180.20 deaths per 100,000 people. The mean hypertension death rate was estimated at 255.31 per 100,000 people (SD = 108.19), ranging from 37.8 to 180.20 deaths per 100,000 people (Table 1).
We used descriptive statistics, factor analysis, and negative binomial models of COVID-19 cases and deaths. We conducted all analyses separately for COVID-19 cases and COVID-19 deaths. Descriptive statistics (mean, standard deviation) were used to describe selected county characteristics. Factor analysis was used to assess whether a smaller number of linear combinations describe the socioeconomic structure of counties in the United States. Negative binomial models were used to examine bivariate associations between concentrated disadvantage, income inequality, racial/ethnic and immigrant concentrations and COVID-19 diagnosed cases and deaths. In multivariate analyses, negative binomial models were also used to assess the effects of those social structural factors on COVID-19 cases and deaths controlling for potential confounders. An interaction term between the proportion of Latinos and the proportion of immigrants was added in those models to determine the effects of Latino immigrant concentration on COVID-19 cases and deaths. The natural logarithm of county population (/100,000) was used as an offset term.
Results
Figures 1 & 2 show the cumulative number of COVID-19 confirmed cases and deaths from January 22, 2020 to August 31, 2020. As of August 31, 2020, close to six million people (5,997,636) had a confirmed diagnosis of COVID-19 and over 180 thousand people (182,169) in the United States died as a result of COVID-19 (Figure 1 & Figure 2).
Figure 1. Cumulative Number of COVID-19 Cases, January, 22 – August, 31 2020
Figure 2. Cumulative Number of Deaths by COVID-19, January, 22 – August, 31 2020
Table 2 displays the unadjusted associations between county concentrated disadvantage, income inequality, racial/ethnic and immigrant concentrations and COVID-19 diagnosed cases. The results show that at the county level, concentrated disadvantage, Gini coefficient, Black concentration, and immigrant concentration were significantly positively associated with higher rates of COVID-19 cases. The incidence rate ratios of COVID-19 cases were 1.327 (95% CI: 1.286, 1.1369) for concentrated disadvantage, 1.301 (95% CI: 1.257, 1.347) for income inequality, 1.237 (95% CI: 1.191, 1.285) for Latino concentration, 1.507 (95% CI: 1.449, 1.568) for Black concentration, 1.063 (95% CI: 1.015, 1.112) for Asian concentration, and 1.263 (95% CI: 1.218, 1.311) for immigrant concentration. In addition, higher rates of COVID-19 cases were associated with chronic disease comorbidities (RR: 1.290, 95% CI: 1.249, 1.333) for chronic disease comorbidities.
Table 2. Unadjusted Negative Binomial Models of COVID-19 infections and deaths.
More specifically for chronic disease comorbidities, the prevalence of obesity and diabetes and cardiovascular disease, stroke, and hypertension death rates were significantly and positively associated with higher rates of COVID-19 cases. The rate ratios of COVID-19 cases were 1.172 (95% CI: 1.135, 1.211) for obesity, 1.282 (95% CI: 1.237, 1.330) for diabetes, 1.234 (95% CI: 1.193, 1.275) for CVD death rate, 1.243 (95% CI: 1.202, 1.286) for stroke death rate, and 1.182 (95% CI: 1.141, 1.225) for hypertension death rate. In addition, higher rates of COVID-19 cases were associated with a greater proportion of uninsured residents (RR: 1.385, 95% CI: 1.332, 1.441), residential mobility (RR: 1.065, 95% CI: 1.028, 1.104), and residence in metropolitan areas (RR: 1.203, 95% CI: 1.119, 1.294). Lower rates of COVID-19 cases were associated with a greater proportion of older people 65 years and older (RR: 0.746, 95% CI: 0.723, 0.770) (Table 2).
Table 2 also displays the unadjusted associations between county concentrated disadvantage, income inequality, and racial/ethnic and immigrant concentrations and COVID-19 deaths. Concentrated disadvantage, income inequality, Latino concentration, Black concentration, Asian concentration, and immigrant concentration were significantly and positively associated with COVID-19 deaths. Higher COVID-19 deaths were associated with greater concentrated disadvantage (RR: 1.214, 95% CI: 1.177, 1.253), higher income inequality (i.e., higher Gini coefficient) (RR: 1.459, 95% CI: 1.403, 1.518), a higher proportion of Latino residents (RR: 1.171, 95% CI: 1.126, 1.219), a higher proportion of Black residents (RR: 1.618, 95% CI: 1.553, 1.686), a proportion of Asian residents (RR: 1.198, 95% CI: 1.143, 1.255), and higher immigrant concentration (RR: 1.262, 95% CI: 1.218, 1.309). In addition, higher rates of COVID-19 deaths were associated with higher chronic disease comorbidities (RR: 1.196, 95% CI: 1.157, 1.236). Higher rates of COVID-19 deaths were associated with obesity (RR: 1.092, 95% CI: 1.055, 1.130), diabetes (RR: 1.257, 95% CI: 1.209, 1.307), CVD (RR: 1.197, 95% CI: 1.154, 1.241), stroke (RR: 1.130, 95% CI: 1.094, 1.167), and hypertension (RR: 1.143, 95% CI: 1.102, 1.187). In addition, higher rates of COVID-19 deaths were associated with a greater proportion of uninsured residents (RR: 1.201, 95% CI: 1.154, 1.249) and residence in metropolitan areas (RR: 1.413, 95% CI: 1.307, 1.527). Lower rates of COVID-19 deaths were associated with residential stability (RR: 0.878, 95% CI: 0.843, 0.914) (Table 2).
Using multivariate analysis, we next examined the effects of concentrated disadvantage (Model 1), income inequality (Model 2), racial/ethnic composition and immigrant concentration (Model 3), and a combined model of social inequality on COVID-19 cases (Model 4), controlling for potential confounders at the county level. The results are displayed in Table 3. After controlling for chronic disease comorbidities, proportion of uninsured residents, proportion of residents 65 years or older, residential mobility, and metropolitan location, the results show that a higher concentration of disadvantage in a county was associated with higher COVID-19 cases (1.227, 95% CI: 1.160, 1.298) (Model 1, Table 3). Higher income inequality in a county was also associated with higher COVID-19 cases (RR: 1.199, 95% CI: 1.155, 1.245) (Model 2, Table 3), net of the effects of potential confounding covariates.
Table 3. Adjusted Negative Binomial Models of COVID-19 Infection Rates.
In addition, after controlling for those covariates, a higher proportion of Latino (RR: 1.106, 95% CI: 1.040, 1.176), Black (RR: 1.379, 95% CI: 1.318, 1.444), and immigrant (RR: 1.565, 95% CI: 1.444, 1.697) residents in a county was associated with higher COVID-19 cases, respectively (Model 3, Table 3). In contrast, a higher proportion of Latino immigrants, an interaction term between the proportion of Latinos and the proportion of immigrants (RR: 0.931, 95% CI: 0.909, 0.953) and Asian concentration (RR: 0.816, 95% CI: 0.775, 0.858) residents were associated with lower COVID-19 cases, respectively (Model 3, Table 3). Finally, after controlling for the effects of potential confounders in the combined model (Model 4, Table 3), higher rates of COVID-19 cases were associated with concentrated disadvantage (RR: 1.082, 95% CI: 1.016, 1.152), income inequality (RR: 1.053, 95% CI: 1.010, 1.098), Latino concentration (RR: 1.091, 95% CI: 1.023, 1.163), Black concentration (RR: 1.336, 95% CI: 1.272, 1.403), and immigrant concentration (RR: 1.580, 95% CI: 1.454, 1.716), whereas lower rates of COVID-19 cases were associated with Asian concentration (RR: 0.814, 95% CI: 0.773, 0.856) and Latino immigrant concentration (RR: 0.925, 95% CI: 0.903, 0.948), respectively (Model 4, Table 3).
Next, we examined the effects of concentrated disadvantage (Model 1), income inequality (Model 2), racial/ethnic composition and immigrant concentration (Model 3), and a combined model of social inequality on COVID-19 deaths (Model 4), controlling for potential confounders at the county level. The results are presented in Table 4. Concentrated disadvantage, income inequality, Latino concentration, Black concentration, and immigrant concentration were significantly positively associated with COVID-19 deaths. After controlling for potential confounders, higher COVID-19 deaths were associated with greater concentrated disadvantage (RR: 1.260, 95% CI: 1.185, 1.339), higher income inequality (RR: 1.393, 95% CI: 1.335, 1.455), a higher proportion of Latino residents (RR: 1.116, 95% CI: 1.041, 1.197), a higher proportion of Black residents (RR: 1.533, 95% CI: 1.459, 1.610), and higher immigrant concentration (RR: 1.629, 95% CI: 1.480, 1.793). In contrast, lower COVID-19 deaths were associated with a higher proportion of Asian residents (RR: 0.842, 95% CI: 0.781, 0.908) and a higher proportion of Latino immigrants (RR: 0.925, 95% CI: 0.902, 0.948), net of the effects of potential confounders (Model 3, Table 4).
Table 4. Adjusted Negative Binomial Models of COVID-19 Death Rates
After controlling for the effects of potential confounders in the combined model (Model 4), higher rates of COVID-19 cases were associated with concentrated disadvantage (RR: 1.102, 95% CI: 1.026, 1.184), income inequality (RR: 1.114, 95% CI: 1.060, 1.171), Latino concentration (RR: 1.096, 95% CI: 1.019, 1.178), Black concentration (RR: 1.448, 95% CI: 1.373, 1.527), and immigrant concentration (RR: 1.634, 95% CI: 1.480, 1.803) whereas lower rates of COVID-19 deaths were associated with Asian concentration (RR: 0.841, 95% CI: 0.780, 0.907) and Latino immigrant concentration (RR: 0.919, 95% CI: 0.895, 0.943), respectively (Model 4, Table 4).
Discussion and Implications
The results imply that existing social inequalities contributed to COVID-19 diagnosed cases and deaths. Four dimensions of county social inequality—concentrated disadvantage, income inequality, racial/ethnic composition, and immigrant concentration—were associated with higher rates of COVID-19 diagnosed cases and deaths. These relationships persisted even after adjusting for the influences of county index of chronic disease comorbidities, encompassing the prevalence of obesity and diabetes, and mortality rates of cardiovascular disease, hypertension, and stroke, and other potentially confounding factors such as the proportion of older (65 years and older) and uninsured residents, residential mobility, and metropolitan location. These findings are consistent with studies that highlight the importance of social and structural determinants of health and health inequalities.11, 12, 13, 14, 15
There are, however, several limitations of the present study. First, this study relies only on county-level data. Individual-level data on COVID-19 cases and deaths by socioeconomic status, race/ethnicity, and immigrant status were not immediately available and are often underreported or not accurately reported in surveillance systems. County-level characteristics tend to be highly correlated and may cause multicollinearity- and confounding-related issues. We ran a series of factor analyses to assess whether a smaller number of linear combinations of county characteristics describe the social structure of those counties. We also controlled for potential confounding factors in the relationships between social structural inequalities and COVID-19 cases and deaths.
Second, the statistical analysis was cross-sectional in design; causal effects of social structural inequities on COVID-19 cases and deaths could not be analyzed across time. Also, beyond the scope of this study, county-level environmental factors, social mechanisms that may mediate the effects of social structural inequities on COVID-19 cases and deaths, most notably social cohesion and social capital, as well as individual-level intermediary mechanisms, including material circumstances, behaviors and biological factors, and psychosocial factors (WHO framework of social determinants of health) were not included in this analysis.
Finally, counties are large spatial entities that may entail greater within- and between-county variations in COVID-19 cases and deaths. An analysis at the neighborhood level, if data were available, would be more informative on the effects of social structural inequalities on COVID-19 cases and deaths.
The COVID-19 pandemic has made clear the structural holes that exist in our social and health systems that affect health and perpetuate health disparities. To address the pandemic, a concerted effort should focus on addressing the upstream social structural inequalities that affect health outcomes. It is time for all stakeholders, especially policy makers, private and non-private organizations and institutions, and community coalitions to work together to address long standing inequities and commit to systematically eliminate all aspects of structural racism. Krouse (2020) put it better this way:
There has never been a more pressing time for us to enact progressive and far-reaching changes in social, economic, and political policies that will shape programs aimed at improving the health of all people living in the United States. We will need to address the larger issues concerning inequities in health for many groups in our country based on decades of inequalities. We need to concentrate on those individuals in lesser health and raise them to the status of healthy. This will involve influencing changes in social, economic, and political decisions and policies that shape national programs affecting our health. We can begin in our own communities, our own hospitals, and our own practices (pp. 1-2).16
Williams and Couper (2020) argue that the U.S. must develop a new kind of “herd immunity,” whereby resistance to the spread of poor health in the population occurs when a sufficiently high proportion of individuals, across all racial, ethnic, and social class groups, are protected from and thus “immune” to negative social determinants.11
References
Endnotes
1Unfortunately, the data from the CDC is not comprehensive, with race/ethnicity available for only 50% of the confirmed cases and 82% for deaths.
2 Centers for Disease Control and Prevention. 2020. Retrieved at: https://covid.cdc.gov/covid-data-tracker/#demographics.
3 Do, D. P., Frank, R., Zheng, C. & Iceland, J. (2017). Hispanic Segregation and Poor Health: It’s Not Just Black and White. Journal of Epidemiology, 86(8), 990-999.
4 Kim, S. J., & Bostwick, W. (2020). Social Vulnerability and Racial Inequality in COVID-19 Deaths in Chicago. Health education & behavior, 47(4).
5 Wadhera, R. K., Wadhera, P., Gaba, P., Figueroa, J. F., Maddox, K. E. J., Yeh, R. W., & Shen, C. (2020). Variation in COVID-19 hospitalizations and deaths across New York City boroughs. Jama.
6 Yancy, C. W. (2020). COVID-19 and African Americans. Jama.
7 Williams, D. R., & Collins, C. (2016). Racial residential segregation: a fundamental cause of racial disparities in health. Public health reports.
8 Williams, D. R., & Mohammed, S. A. (2009). Discrimination and racial disparities in health: evidence and needed research. Journal of behavioral medicine, 32(1), 20-47.
9 Pirtle, W. N. L. (2020). Racial capitalism: A fundamental cause of novel coronavirus (COVID-19) pandemic inequities in the United States. Health Education & Behavior, 1-5.
10 Steven Manson, Jonathan Schroeder, David Van Riper, and Steven Ruggles. IPUMS National Historical Geographic Information System: Version 14.0 [Database]. Minneapolis, MN: IPUMS. 2019. http://doi.org/10.18128/D050.V14.0
11 Diez Roux, A. V. (2012). Conceptual approaches to the study of health disparities. Annual review of public health, 33, 41-58.
12 Link, B. G., & Phelan, J. (1995). Social conditions as fundamental causes of disease. Journal of health and social behavior, 80-94.
13 Phelan, J. C., Link, B. G., & Tehranifar, P. (2010). Social conditions as fundamental causes of health inequalities: theory, evidence, and policy implications. Journal of health and social behavior, 51(1_suppl), S28-S40.
14 Williams, D. R., & Sternthal, M. (2010). Understanding racial-ethnic disparities in health: sociological contributions. Journal of health and social behavior, 51(1_suppl), S15-S27.
15 Diez Roux, A. V., & Mair, C. (2010). Neighborhoods and health.
16 Krouse, H. J. (2020). COVID-19 and the Widening Gap in Health Inequity. Otolaryngology–Head and Neck Surgery, 0194599820926463.
Appendix A. Principal Component Factor Analysis